

Specialist Analytics

Modules dedicated to specialist analytics
Data-driven client segmentation
Understanding client characteristics and segmenting clients based on their similarities is an important task in banking. Having a solid client segmentation program enables systemic, uniform, and strategic management of clients – making business optimization feasible. It allows for many business applications like pricing, fee structures, strategic tuning, dedicated campaigns, special risk, and potential considerations and so on.
Understanding client characteristics and segmenting clients based on their similarities is an important task in banking.
Having a solid client segmentation program enables systemic, uniform and strategic management of clients – making business optimization feasible. It allows for many business applications like pricing, fee structures, strategic tuning, dedicated campaigns, special risk, and potential considerations and so on.
Banks have many thousands or even millions of clients that need to be included in the segmentation. To determine common client characteristics a set of homogeneity parameters are selected - often these include information about client type, profitability, age, credit quality, future potentials etc.
The analytical challenge is to optimally segment many thousand clients into a number of homogeneous segments based on the selected client homogeneity parameters. The application helps do exactly that.
Some questions to answer:
- Should there be an overarching segmentation structure, e.g. different for privates and corporates?
- Which homogeneity parameters to use for segmentation?
- How many segments are required to get a high degree of homogeneity?
- How are segments defined in terms of homogeneous parameters?
- Which segment do individual clients belong to?
The AQBanking solution suite provides advanced unsupervised learning methodology to help answer all these questions and includes supportive visualizations.
The client segmentation is performed in a matter of seconds and results are readily available for exporting.
Read more
Visualizing client characteristics
Having many thousands or even millions of clients it can be a difficult task to thoroughly understand differences and shared characteristics across clients.
Having many thousands or even millions of clients it can be a difficult task to thoroughly understand differences and shared characteristics across clients.
The AQBanking solution suite helps take a deep dive and visualize the characteristics across all and subsets of clients for any relevant business variables the user sees fit.
Some examples - understand and visualize the characteristics for...
- Clients that have defaulted
- Clients in a given segment
- Clients in a given credit rating
- Clients in a given age group
- Clients that failed credit granting
The application provides unique and advanced visualization to provide a full break-down of client characteristics. It makes it easy to detect special patterns, outliers, likely data errors or undesired properties.
The visualizations are provided in seconds and are readily available for exporting.
Read more
Credit quality migration
Understanding how credit quality has migrated over a period is of high importance in banking and it helps understand how the riskiness of the credit portfolio has evolved and whether or not credit quality has improved or deteriorated.
The solution helps break down rating migration for a user-defined period, like the year 2021 or the period 2018-2021. Typically, 1-year periods are applied when estimating transitions matrices.
Some of the results across 1) the total credit portfolio, 2) privates/corporates and 3) individual sectors include:
- Count and volume-based one-year transition matrices including empirical default rates
- Multi-year transition matrices including probability of default
- Count and volume-based rating distributions of new clients on the books
- Count and volume-based rating distributions of leaving clients
Reporting rating migration is relevant in all banks with credit exposure and includes tasks that often require significant efforts and time consumption. The application solves this in just seconds and results are supported by innovative visualizations that allow for results that are easy to communicate.
The results of the application are used keep a continuous understanding of the overall migration of credit quality and is furthermore often used for back-testing PD models.
Read more
Long term credit quality cycles
Understanding the development of credit quality across (long term) credit cycles and where you currently are in the cycle are important input to optimally perform strategic credit portfolio management.
Just as it is important to understand credit migration for a fixed (often 1-year) period, it has high business value to understand how the credit quality has evolved on portfolio levels over longer horizons and how it is trending right now. Whether or not credit quality trends for improvement and deterioration should impact management decision-making related to risk appetite and credit granting.
The application helps break down the development and index credit quality across time. Often quite extensive time horizons - sometimes even full credit cycles - are applied to better understand and monitor how credit quality has changed with macroeconomic conditions.
The AQBanking solution suite shows the development of credit quality in terms of number of clients and volume across:
- The entire credit portfolio
- Privates and corporates
- Individual sectors
Results are available in seconds and provides both numerical tables and supportive visualizations.
Read more
Credit concentrations
Managing concentration risk is of key importance in banking. Everybody knows not to put all their eggs in one basket – that’s basically what managing concentration risk is all about. Although it seems basic, concentration risk has been on the forefront and a fundamental driver in many financial crises.
Concentration risk arise in different shapes and typically credit concentration is broken into the following categories:
- Single name concentration – large exposures to single clients.
- Sector concentration – large exposures to individual sectors
- Geographical concentration – large exposures in individual regions
- Collateral concentration – large exposures to the same or same type collateral
The reasoning behind considering sector concentrations is that different companies in the same sector are exposed to many of the same systemic risk factors and so if one company is in trouble due to systemic reasons – e.g., decreasing prices, increased costs etc., most likely, other companies in the same sector is dealing with the same issues and therefore may also be in trouble.
The same type of reasoning can be made for geographic concentration where local systemic factors, like weather, employment rate etc., may impact companies and privates similarly within the same region.
Most collateral is typically provided from real estate and if the value of real estate decreases it is often systemic and it impacts the size of non-collateralized exposures and thus risk significantly.
Excessive concentrations dramatically increase the probability of large losses, as just a one or few defaults or systemic changes need to happen before it significantly impacts banks. Capital requirements should therefore go up when concentrations arise, however the regulatory portfolio capital methods explicitly assume that credit portfolios are perfectly diversified (i.e. zero concentration). To account for that ad-hoc measures to monitor and put a limit on sector concentrations have been imposed using the Herfindahl–Hirschman Index (HHI). The benefit of the HHI measure is its simplicity - it has known weaknesses, but it does to some degree account for sector concentrations.
The AQBanking solution suite performs a full break down of concentrations across single names, sectors, geography and collateral. It also provides the sector HHI key-ratios for the regulatory reporting.
Results are available in seconds and provides both numerical tables and supportive visualizations.
Read more
Credit parameters
When performing credit analyses and credit risk calculations several credit parameters are needed. The AQBanking solution suite supports estimating these parameters empirically or based on statistical models.
Probability of Default
Probability of Default (PD) reflects the probability that a client will default in the coming year.
Based on banks own default/non-default experience of clients and desired model variables - the application provides a logistic regression framework, that estimates PDs for each client.
The framework includes a lot of supportive model assessment utilities, like visualization of input variables, cross-table functionality and model accuracy functionality like the ROC curve and the resulting client PD distributions across rating classes etc. are shown.
Client PDs is easily exported to excel.
Loss Given Default
Loss Given Default (LGD) is the expected loss ratio when clients default. For instance, LGD of 10% means that 10% of the exposure at default is lost.
Distributions of LGD values are often bimodal, so either LGD values are low or high.
The AQBanking solution helps assess appropriate LGD levels across sectors based on empirical data. Statistics for LGDs across sectors are provided and LGD distributions are available graphically.
Credit Conversion Factor
The Credit Conversion Factor (CCF) is related to credit facilities and express how much clients are assumed to utilize credit lines, when credit quality deteriorates.
CCF also has regulatory significance as it in a regulatory context can take values of: 0, 20, 50 or 100% depending on the duration of notice required to cancel the credit facility.
The application estimates actual empirical CCFs experienced from clients and across credit products. Statistics are provided for each credit product and supportive distributions are available. CCF distributions are typically fat-tailed weighted toward 100%, i.e., full utilization.
Collateral hair-cuts
Hair-cuts on collateral values reflect the expected reduction in value when having to realize the asset, e.g. due to liquidity issues. Hair-cuts can be estimated empirically for the most common types of collateral, like real-estate.
The application estimates empirical hair-cuts from experienced collateral realizations and across the different types of collateral – it also considers differences across geographic regions.
Read more
Risk and capital adequacy calculations
The AQBanking solution suite supports internal portfolio credit risk assessments. The risk calculations include several advanced portfolio risk models that are simulation or analytical based and includes wide modelling flexibility. The internal models automatically handle concentration risk appropriately.
The risk calculations include a flexiblestress testing framework where credit risk parameters and correlations can beshocked and portfolio level consequences evaluated. Ten stress tests can beexecuted simultaneously.
The portfolio risk calculations and stress can be used as framework for the internal capital adequacy assessment process (ICAAP). The application also includes the foundation and advance regulatory IRB portfolio capital models.
Having capital results from the standard approach, the fIRB and aIRB approaches and full portfolio credit risk models as well as stress testing, provides comprehensive input to the ICAAP assessments and reporting can be utilized from the AQBanking solution suite.
Throughout the solution extensive visualizations support analysis and result interpretation.
Read more
Explore other solutions
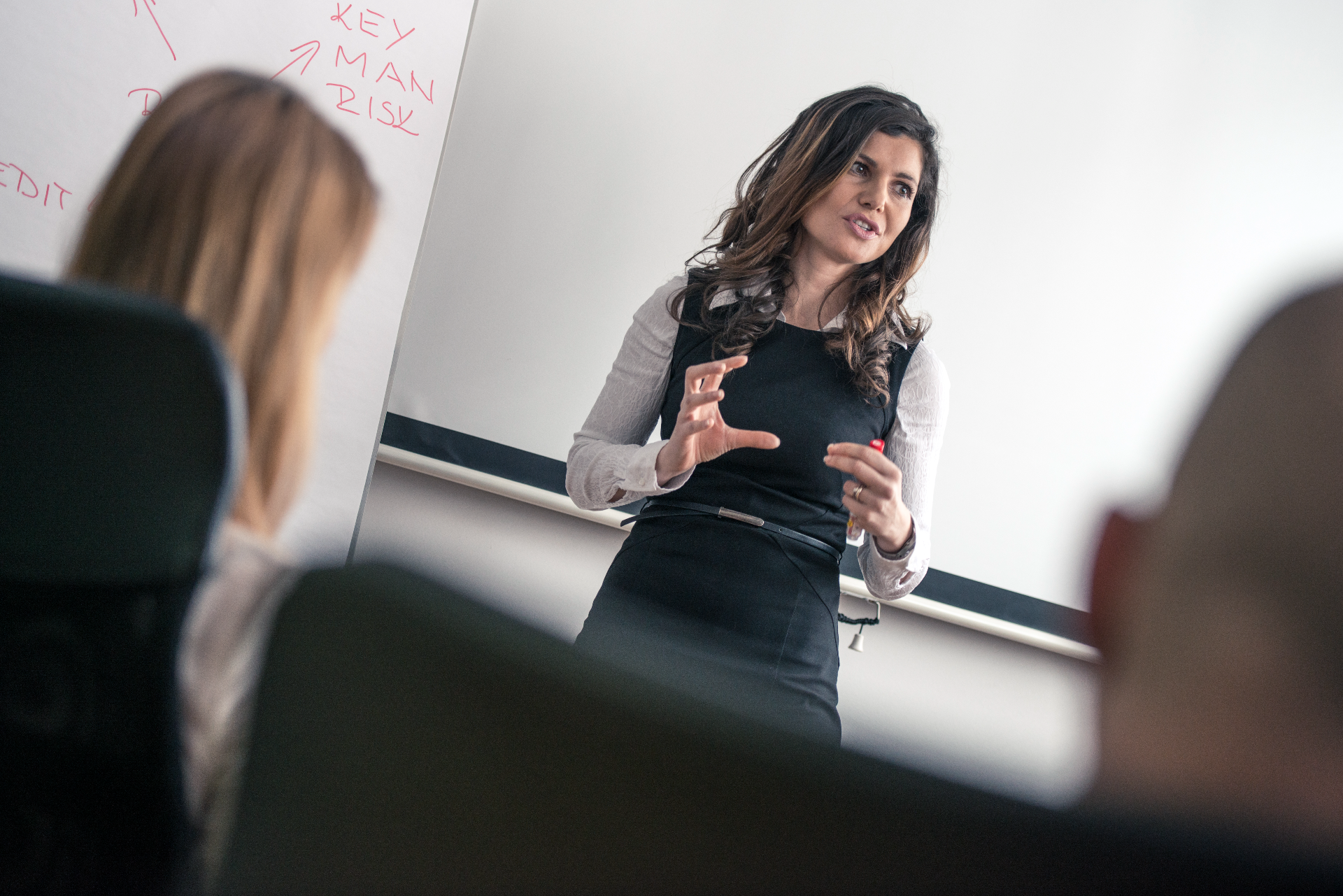
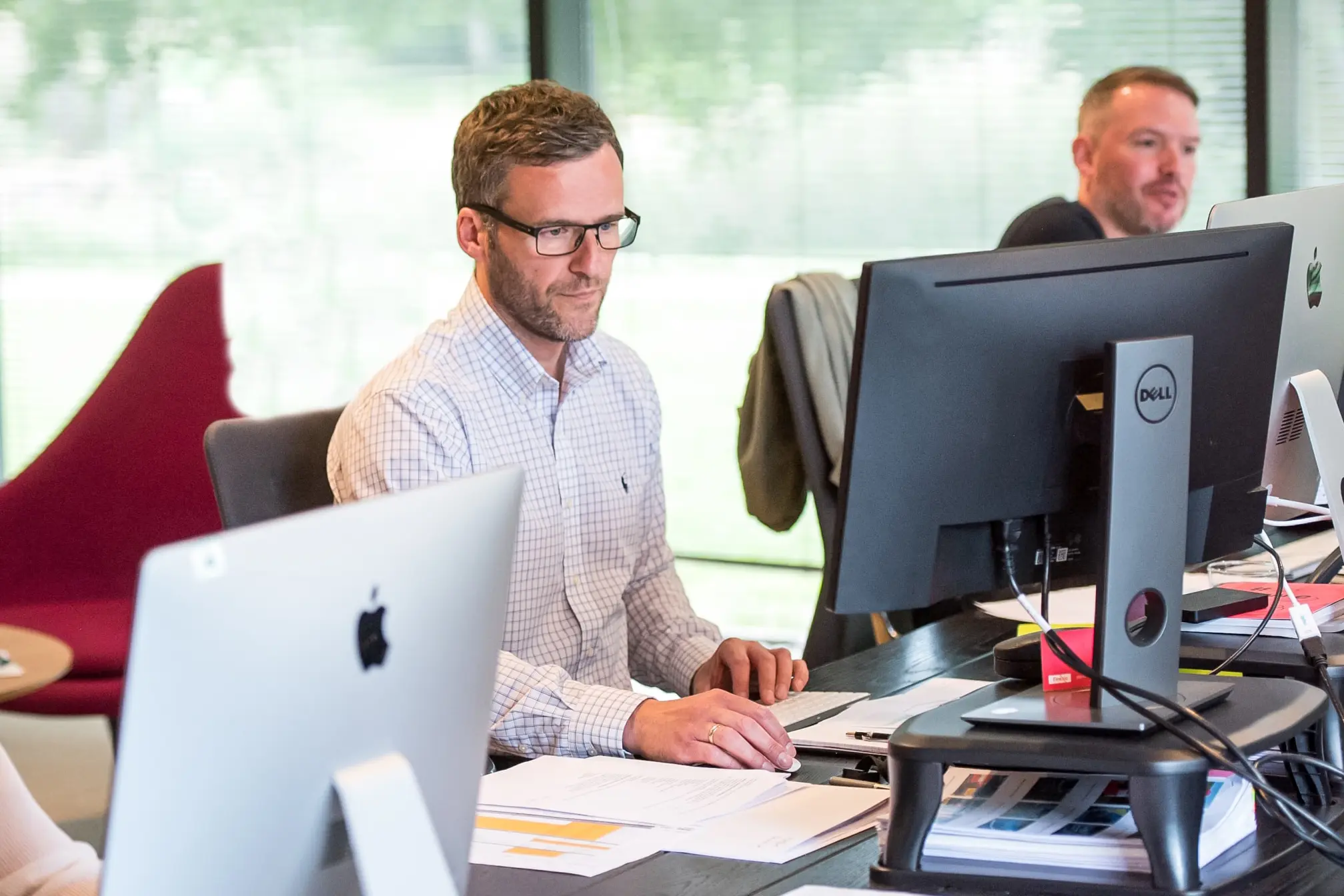